In a previous blog, we already uncovered some of the magic behind machine learning. Today, we take you on a deeper dive into this fascinating topic.
Eye of the tiger
Our bird identification model functions based on the principle of deep learning. For the machine to effectively “see” what is in the photograph, it makes use of three models, each with a higher degree of specificity, to help us get to the finish line: determining the species.
To start, what you need is a lot of data or, in our case, images of birds. We obtained those via a worldwide network of some 100 test cameras that some of our backers were kind enough to set up and manage. Another source of images with which we trained our species identification model were submissions from bird-loving volunteers. In total, millions of images were received and classified.
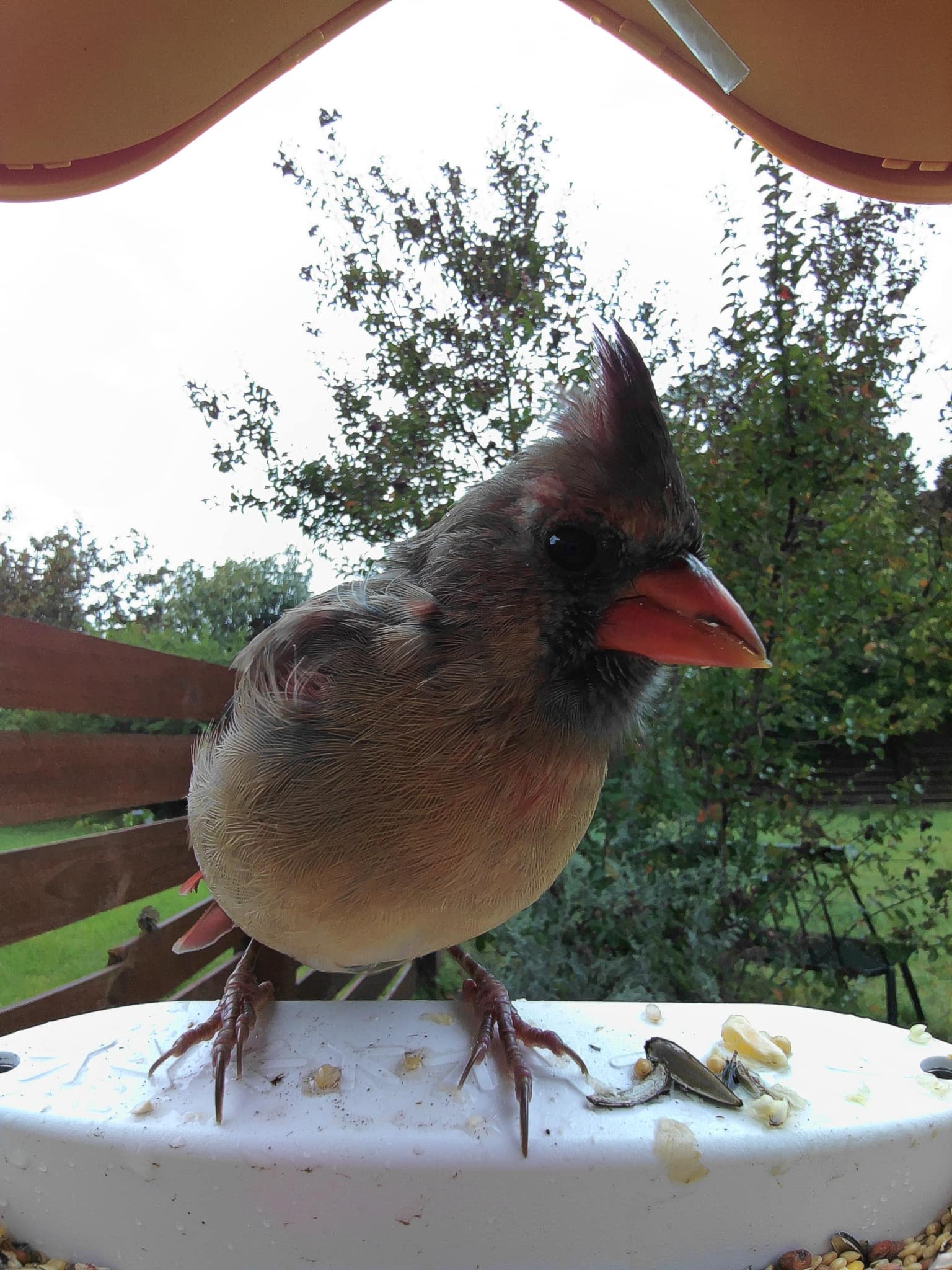
Practice makes perfect
Before an identification model can be created, it must first be trained with the highest possible amount of data of the highest quality. Better quality translates to higher accuracy. The very first stage in the training process is actually done manually. There is an entire team of labelers dedicated to determining where the bird or birds are in the photograph and assigning the respective species.
Once the data the model is going to be fed is prepared, training begins. You can visualize the process of training itself as a game of connect the dots: we have a certain amount of images of, say, a blue tit available – these are the dots. We know what we want our output to be: we want the model to identify them as a blue tit – this is the final image in which the dots are connected. When we feed all the tagged images of a certain species into our model, it will memorize certain characteristics and features to later correctly identify the bird. This will be done in the so-called inference phase, once the model has been adequately trained and exported.

Now you see me, now you don’t
After the model has been trained using millions upon millions of images, not to mention the dedicated skills of the team responsible for tagging them, all of the things the model has learnt are frozen in place and the model exported. Now, it is ready to identify your neighborhood pals.
The identification process, as stated, consists of three phases. In the first phase, images are divided into “interesting” and “not interesting” ones. By interesting, we mean images that can be useful for determining the species of a bird, not ones where a blue jay is striking a particularly dramatic pose! Interesting images are, therefore, images in which a bird is in the frame and in focus, and in which at least one eye and the beak are visible; those, in short, that are worthwhile analyzing. Funnily enough, though, images that we would find subjectively interesting – think of the aforementioned blue jay – are the ones that the model has the easiest time identifying!
One bird, two birds, three birds…
The second model is called a detector: it detects how many individual birds are present in the image and singles it out by creating a bounding box around it: this process is known as object localization. Each of these detected birds are analyzed by the deep learning model that returns an estimate of how likely it is that the bird in question belongs to one species or another. The end result looks a little something like this:
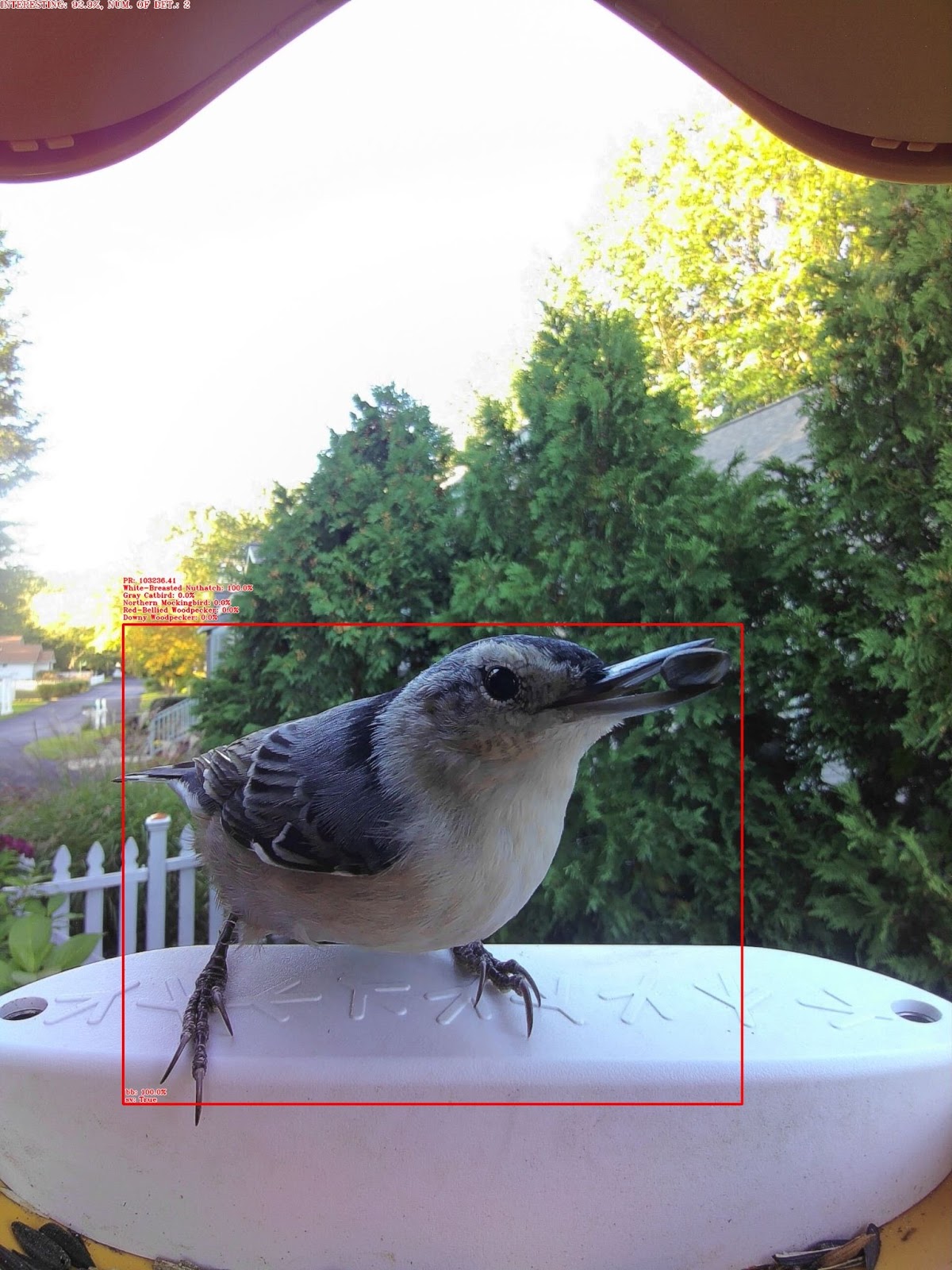
Here, thanks to deep learning, the white-breasted nuthatch was identified with 100% accuracy! Way to go! We have mentioned the term deep learning a few times, so what exactly is deep learning and how does it determine which species of bird has just paid you a visit?
Going on a deep dive
When you use a deep learning approach, you teach a machine to learn by example: for instance, by feeding it many photos of a certain bird. Deep learning models are composed of something called a neural network, at least most of them are, which is why another name for them is also a deep neural network or DNN.
Neural networks, thus called because the inspiration for their structure was derived from the human brain, are extremely good at one thing: pattern recognition. A deep neural network consists of three layers: the input layer – the image of a cool bird perched on your feeder –, hundreds of hidden layers – how many there will be is determined in the training process –, and the output layer – this provides the estimate of how likely it is that your visitor is a white-breasted nuthatch.
The unknown
Here is where things get interesting: in contrast to machine learning that involves manually selecting which specific features are characteristic of a particular type and feeding that into a model, a deep learning model does all that by itself. When it is fed images, it extrapolates hundreds of characteristics that, in the end, help it decide on one species instead of another.
Which are they? Why is this a tit and not a warbler? Well… We just don’t know! While the math on deep learning models is crystal clear, the hidden layer, within which all of this information gets analyzed and cross-referenced, is precisely that: hidden. So, though we may know what happens and how it happens, we are simply unable to tell what exactly leads the model to draw a specific conclusion. It just knows. That’s all.